20. prosince, v rámci očekávané akce OpenAI 12 dní Vánoc, společnost představil model o3-nová rodina modelů uvažování, která je připravena nově definovat schopnosti umělé inteligence.
Toto oznámení vyvolalo v komunitě odborníků na umělou inteligenci šok, protože o3 vykazuje nebývalý výkonnostní skok v mnoha benchmarcích, což naznačuje, že se umělá inteligence blíží k umělé obecné inteligenci (AGI). Model o3 vyniká nejen jako iterace, ale jako hluboký posun v architektuře AI, která je schopna uvažovat způsobem napodobujícím řešení lidských problémů.
Nejde jen o malou aktualizaci. o3 představuje zásadní posun v architektuře umělé inteligence a nabízí schopnosti uvažování, které napodobují lidské řešení problémů způsobem, jaký jsme dosud neviděli.
Dnes jsme se podělili o hodnocení rané verze dalšího modelu v naší sérii o-modelových úvah: OpenAI o3 pic.twitter.com/e4dQWdLbAD
- OpenAI (@OpenAI) 20. prosince 2024
Rekordní výkon na ARC-AGI
OpenAI o3 dosáhla 75.7% na Polosoukromý benchmark ARC-AGI-skok před GPT-4o's 5% na začátku letošního roku. S větším výpočetním výkonem to společnost o3 posunula ještě dál. 87.5%. To představuje výrazný skok oproti předchůdci o1.
Takový výkon má však svou cenu. Provoz o3 v režimu vysoké účinnosti na 400 veřejných skládačkách ARC-AGI stojí $6,677. Dosažení nejvyššího možného skóre vyžadovalo 172krát více výpočtů, což mohlo vést k dosažení $1,14 milionu pro stejný úkol.
Srovnávací test ARC-AGI je jedním z nejnáročnějších testů umělé inteligence, jehož cílem je posoudit schopnost umělé inteligence řešit hádanky, které jsou pro člověka intuitivní, ale pro stroj náročné. Předchozí modely, jako je GPT-4, narazily na výkonnostní strop při nižších procentech, což zdůraznilo omezení konvenční umělé inteligence. úspěch o3 signalizuje novou hranici, která přesahuje rozpoznávání vzorů a přechází ke skutečnému řešení problémů.
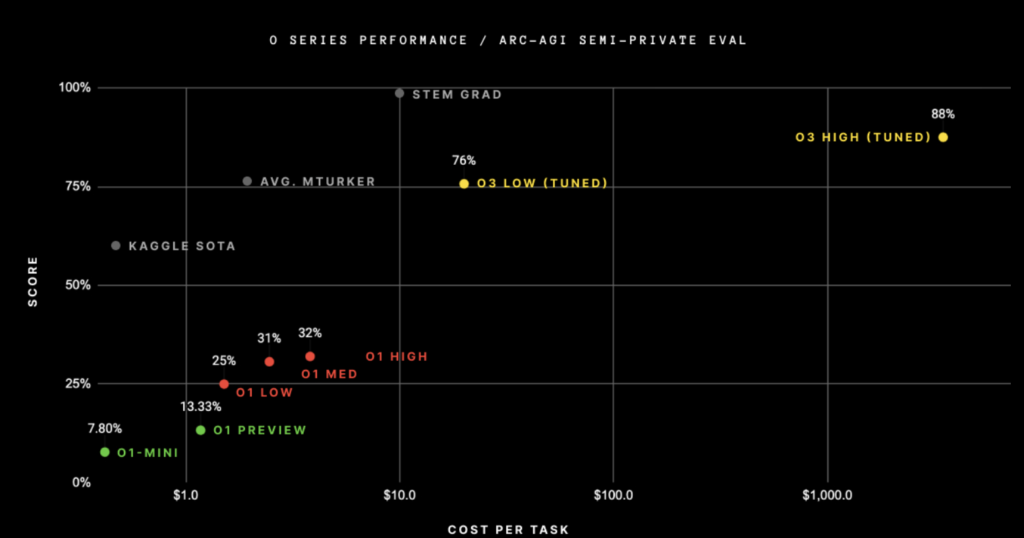
Sam Altman však tvrdí, že o3-mini překonává o1 v mnoha kódovacích úlohách při nižších nákladech, což poukazuje na zvětšující se rozdíl mezi nárůstem výkonu a rostoucími náklady. Tento posun by mohl zvýšit zájem o menší a výkonné modely namísto dražších a větších.
zdánlivě poněkud ztracené v hluku dnešní doby:
- Sam Altman (@sama) 21. prosince 2024
v mnoha kódovacích úlohách o3-mini překoná o1 při obrovském snížení nákladů!
Očekávám, že tento trend bude pokračovat, ale také že možnost získat nepatrně vyšší výkon za exponenciálně více peněz bude opravdu zvláštní.
Jak o3 funguje
o3 je součástí "o-série" společnosti OpenAI, která navazuje na dřívější model o1. Na rozdíl od tradičních modelů GPT, které se zaměřují na generování textu, o3 zavádí syntéza programů řízená hlubokým učením.
To umožňuje modelu dynamicky generovat a testovat nové programy - podobně jako lidé řeší problémy zkoušením různých přístupů. Spojením symbolického uvažování s hlubokým učením se model o3 posouvá od pasivního generování výstupů k aktivnímu řešení problémů.
Klíčové odlišnosti:
- Syntéza programu: o3 nezajišťuje pouze načítání dat. Aktivně konstruuje a iteruje řešení a testuje je, dokud se neobjeví nejlepší odpověď.
- Výpočetní škálování: o3 přizpůsobuje využití výpočetního výkonu v závislosti na složitosti úlohy. Režim nízkého výkonu již překonává předchozí modely, ale režim vysokého výkonu posouvá jeho hranice ještě dále.
- Rozumění v přirozeném jazyce: o3 vysvětluje svá řešení srozumitelným jazykem a nabízí postupné informace o tom, jak dospěl k určité odpovědi.
Referenční úspěchy
Kromě ARC-AGI prokázala společnost o3 dominantní postavení v celé řadě oblastí:
- SWE-Bench Ověřeno: 71,7% (nárůst o 22,8 bodu oproti hodnotě o1), což odráží špičkový výkon v oblasti softwarového inženýrství.
- Codeforces: 2727 Elo - překonává většinu lidských konkurentů v soutěžním programování.
- GPQA Diamond: 87.7% - vynikající výsledky v komplexních vědeckých otázkách na úrovni absolventů.
- Hraniční matematika: 25,2% - překonání předchozího výkonu AI v pokročilé matematice (kde žádný jiný model nedosáhl vyššího skóre než 2%).
Tyto úspěchy ukazují, že aplikace o3 by se mohly rozšířit napříč průmyslovými odvětvími, od automatizace vědeckého výzkumu až po vývoj sofistikovaného softwaru. Vysoké náklady na výpočetní techniku však nadále omezují její širší komerční nasazení.
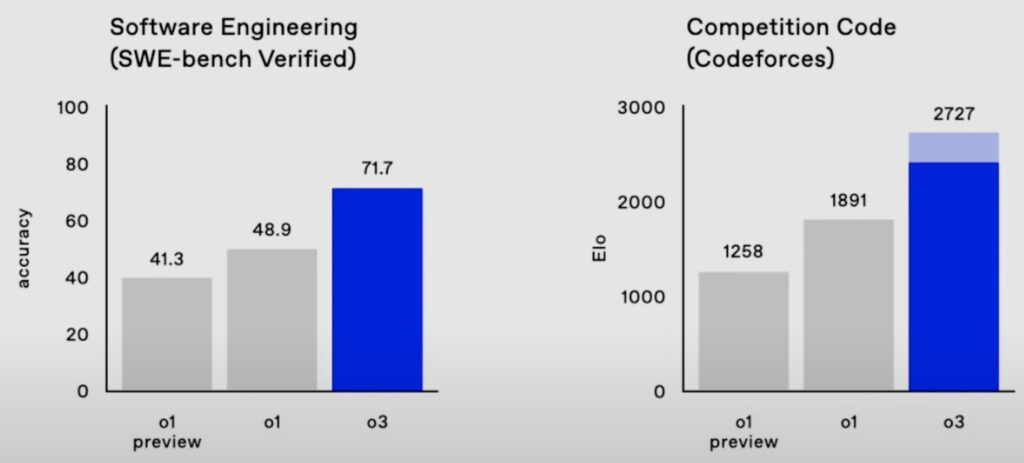
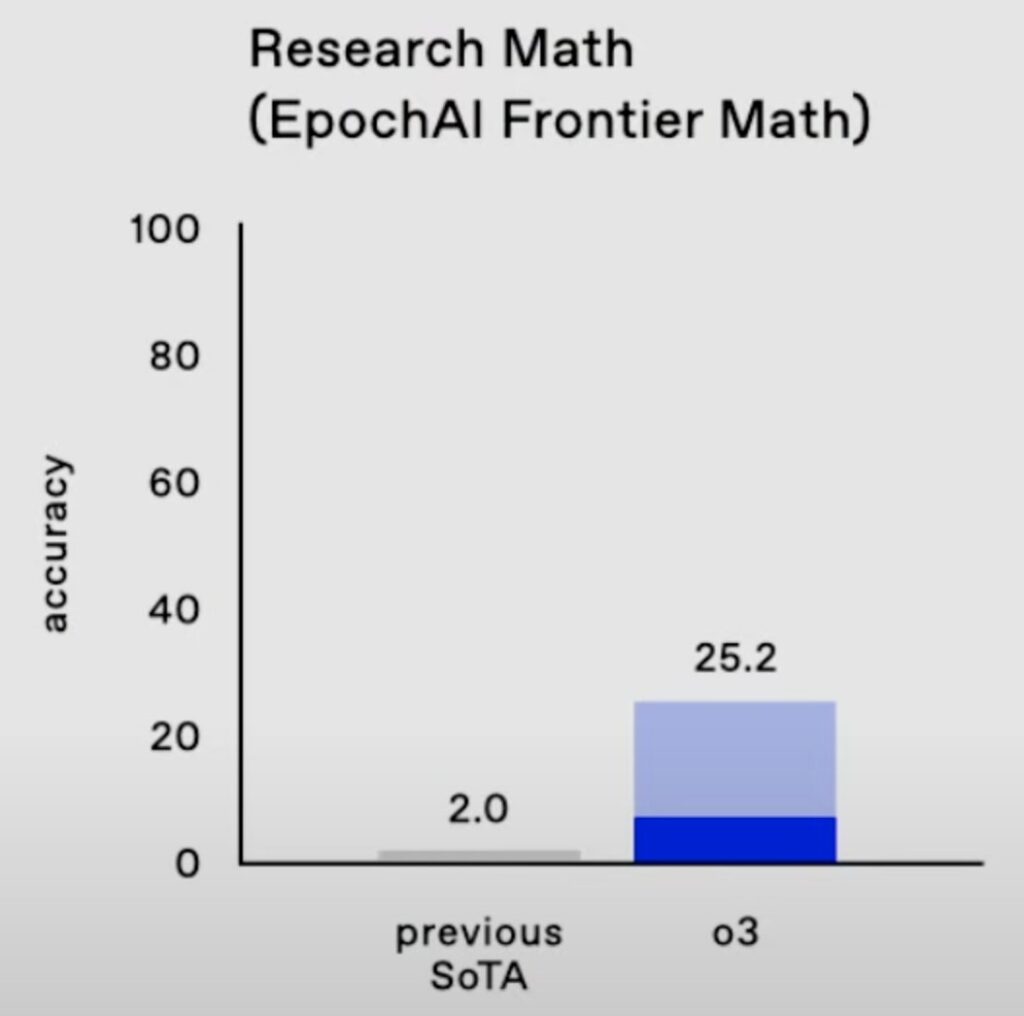
AGI nebo jen krok blíže?
Navzdory působivým pokrokům varují generální ředitel OpenAI Sam Altman a tvůrce ARC-AGI François Chollet před označením o3 AGI.
Zatímco ve strukturovaných úlohách o3 exceluje, v jednodušších úlohách, jako je vizuální rozpoznávání vzorů nebo základní aritmetika s abstraktními symboly, stále naráží. Tyto úlohy zůstávají pro člověka druhou přirozeností, ale i pro nejlepší modely umělé inteligence jsou složité.
Silnou stránkou o3 jsou složitá prostředí založená na pravidlech. Dosažení AGI však bude vyžadovat překonání těchto slabin a vývoj systémů, které budou schopny pružně pracovat v nestrukturovaném reálném prostředí.
Přesto mnozí přirovnávají o3 k "AlexNet moment pro syntézu programu,", což znamená začátek nové éry schopností umělé inteligence. Je to významný milník, ale ne konečná meta pro AGI.
Efektivita vs. výkon
Jednou z největších výzev u systému o3 je efektivita. Při vysokých výpočetních úrovních může každá úloha stát více než $1,000-někdy překračuje $6,000 pro větší benchmarky-a řešení zabere hodně času. 🤯 Tato vysoká cena znamená, že o3 je v současnosti praktičtější pro velké technologické společnosti, vlády nebo výzkumné instituce s vysokým rozpočtem, které řeší složité problémy. Naproti tomu lidské řešení problémů zůstává výrazně levnější a rychlejší.
Prostředí se však rychle vyvíjí. Stejně jako u všech technologií se očekává, že náklady na výpočetní techniku AI se budou snižovat a efektivita se bude s budoucími iteracemi pravděpodobně zvyšovat. Dlouhodobým cílem OpenAI je tyto náklady snížit a zpřístupnit tak uvažování pomocí AI širšímu okruhu průmyslových odvětví, startupů i jednotlivých výzkumníků.
Nadace ARC Prize Foundation se zavázala, že soutěž o hlavní cenu bude probíhat do doby, než bude vyhodnocen vysoce efektivní model s otevřeným zdrojovým kódem. 85% na ARC-AGI. Očekává se, že tato snaha o efektivitu bude určovat budoucí směry výzkumu s důrazem na nákladově efektivní obecnou inteligenci a praktické aplikace umělé inteligence.
Závěr
Model o3 společnosti OpenAI představuje velký skok v oblasti umělé inteligence, který zvyšuje konkurenci mezi společnostmi Microsoft, Google, Apple, Meta a Amazon. Tento závod o AGI urychluje vývoj a posouvá AI blíže k výkonu na úrovni člověka.
Společnost Nvidia těží z toho, že poptávka po grafických procesorech prudce roste, aby mohla pohánět výpočetně náročné modely, jako je o3. Vzhledem k prudce rostoucím nákladům společnosti rozšiřují infrastrukturu, zatímco AMD, Intel a startupy jako Cerebras vyvíjejí efektivnější čipy.
Automatizace umělé inteligence ohrožuje pracovní místa v oblasti softwarového inženýrství, analýzy dat a kreativních oborů. Nejvíce ohroženy jsou pozice na úrovni začínajících pracovníků, zatímco poptávka po specialistech na umělou inteligenci roste. Rychlejší zavádění závisí na snižování výpočetních nákladů a zvyšování efektivity, což by mohlo změnit ekonomiku a donutit vlády, aby přehodnotily politiku zaměstnanosti.
Závod AGI bude pokračovat. Od léčby nemocí až po vědecké objevy - přínosy jsou hnacím motorem. Se zrychlujícími se inovacemi bude umělá inteligence nebývalou rychlostí přetvářet průmyslová odvětví a společnost.