ByteDance, best known as the parent company of TikTok, has just introduced OmniHuman-1, a brand
OmniHuman-1 leverages a large-scale diffusion-based architecture, enabling it to capture natural motion from minimal inputs such as static images, audio signals, or reference videos. As a result, the model opens up possibilities for content creators, filmmakers, and marketers looking to produce captivating digital avatars or fictional video clips with relative ease.
Why OmniHuman-1 Matters
Previous AI-based approaches often fell short when it came to generating complete, coherent human movement. They would typically animate only the face or upper body, leaving body language and gestures looking awkward or inconsistent. OmniHuman-1, however, addresses these issues by presenting fluid, full-body motion that adapts to the user’s input signals.
Furthermore, the model scales gracefully to different aspect ratios and body proportions. It’s not locked to portrait-only perspectives, as some earlier systems were. By accommodating a wide range of formats, it becomes a versatile tool for various media projects, from short-form mobile videos to cinematic widescreen productions.
Technical Foundations
OmniHuman-1 is powered by cutting-edge AI architecture designed to handle complex motion and video generation. This innovative system leverages a combination of diffusion models and multimodal training techniques to create highly realistic human animations.
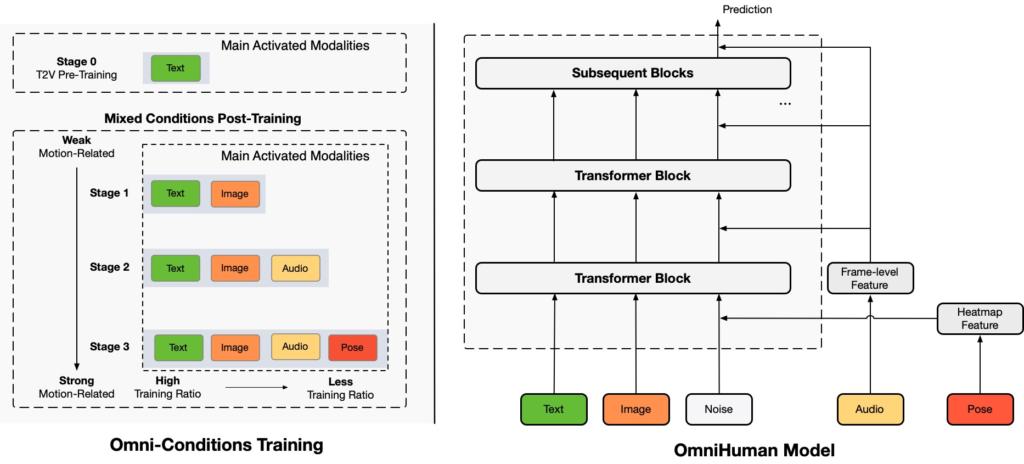
Detailed insights into OmniHuman-1’s architecture, training strategy, and performance are outlined in its official research paper, published on arXiv under the title OmniHuman-1: Generating Human Videos from a Single Image and Motion Signals.
Let’s break down how these components work together to push the boundaries of AI-generated video.
Diffusion Transformer (DiT)
Central to OmniHuman-1’s performance is a Diffusion Transformer (DiT). Diffusion models have already proven effective in creating high-quality images and videos. When combined with the Transformer architecture, they can pay attention to multiple elements over time, handling both local details (like facial features) and global context (like overall body movement).
Omni-Conditions Training
A key part of OmniHuman-1’s training strategy is incorporating “omni-conditions,” which means it blends strong signals—like exact pose data—and weaker signals—like audio or text prompts—during training. This approach allows the model to retain and learn from a broader dataset, rather than discarding videos that don’t have perfect reference annotations. As a result, it develops a more generalized understanding of motion.
19,000-Hour Dataset
OmniHuman-1’s scale is another reason it performs so well. ByteDance researchers report using 19,000 hours of videofor training. Such extensive exposure to varied body movements and scenarios gives it the resilience to produce realistic footage even with unusual poses or camera perspectives.
Key Features
- Single-Image to Video: One highlight is generating a moving, speaking, gesturing subject from just a single image. The user provides a photo plus an audio track, and the model outputs a full-body video that syncs lip movements, gestures, and body language to the speech.
- Multiple Motion Inputs: OmniHuman-1 accepts different motion signals. Audio-driven animation focuses on speech gestures and lip-sync, while video-driven animation replicates or modifies motion from reference footage. A user can even combine these modalities, using audio for speech and a reference clip for upper-body gestures or dance steps.
- Aspect Ratios and Body Proportions: Whether you need a portrait clip for social media or a widescreen video for film, OmniHuman-1 can adapt. It also handles different body shapes and proportions, adding to its versatility in stylistic or narrative contexts.
- Style Adaptations: OmniHuman-1 isn’t restricted to photorealism. It can produce cartoon-like animations, stylized figures, or even anthropomorphic characters. For those in gaming, virtual influencers, or creative filmmaking, the option to switch between styles is especially appealing.
Performance Metrics
ByteDance has provided several benchmarks comparing OmniHuman-1 to other popular AI animation methods (like Loopy, CyberHost, and DiffTED). Here are some notable points:
- Lip-Sync Accuracy (higher is better):
- OmniHuman-1: 5.255
- CyberHost: 6.627
- Loopy: 4.814
- Fréchet Video Distance (FVD) (lower is better):
- OmniHuman-1: 15.906
- Loopy: 16.134
- DiffTED: 58.871
- Gesture Expressiveness (HKV):
- OmniHuman-1: 47.561
- CyberHost: 24.733
- DiffGest: 23.409
- Hand Keypoint Confidence (HKC) (higher is better):
- OmniHuman-1: 0.898
- CyberHost: 0.884
- DiffTED: 0.769
OmniHuman-1 delivers strong performance across all key benchmarks. It excels in overall video quality with the lowest Fréchet Video Distance and achieves the best scores for gesture expressiveness and hand keypoint confidence, indicating natural, precise animations. While its lip-sync accuracy is slightly behind CyberHost, it still ranks competitively in this category.
Limitations
Although OmniHuman-1 is a major step forward, it does face challenges. Low-resolution or poor-quality input images can lead to unnatural poses or blurry facial details in the output video. Extremely complex or rare motion sequences, such as acrobatic stunts, may also produce distortions or artifacts. On top of that, training and running the model require significant computational resources, which could limit accessibility.
Use Cases
OmniHuman-1 opens up new creative possibilities across industries. From digital storytelling to virtual training, this section highlights how different fields can leverage the model’s ability to generate lifelike videos from minimal input.
Digital Content Creation
OmniHuman-1 can revolutionize content production for social media and advertising. Influencers and marketers often need fast, eye-catching video content, and this model allows them to transform a single image into multiple, dynamic video clips. From creating personalized avatars to animating product demonstrations, the technology reduces production costs and complexity without sacrificing creativity or visual quality.
Brands can quickly generate region-specific content by modifying details like gestures, clothing, or backgrounds to suit local preferences. Similarly, music artists could produce animated music videos featuring themselves or fictional characters performing choreographed routines, broadening creative possibilities for digital campaigns and promotions.
Film and Animation
Traditional animation workflows, especially those involving full-body motion capture, can be time-consuming and expensive. OmniHuman-1 offers a powerful alternative by automating complex movements while still allowing directors and animators to maintain artistic control. The model can generate lifelike or stylized character animations, which can be refined further if necessary, shortening post-production timelines.
For experimental filmmakers and indie creators, this opens the door to producing films with high production value on a lower budget. OmniHuman-1’s flexibility in adapting to different art styles also enables hybrid productions that blend realism with fantasy or abstract visuals.
Education and Virtual Storytelling
OmniHuman-1 can make education and storytelling more immersive by animating historical figures or fictional characters from portraits or photographs. Museums and educational institutions could use it to bring exhibits to life, offering interactive experiences where historical personalities deliver lectures or narrate events.
For online learning, animated instructors could enhance engagement in virtual classrooms by providing visual demonstrations of concepts or stories. Storytellers and content creators for children’s media can also generate animated characters that maintain a sense of realism, enhancing both entertainment and learning outcomes.
Virtual Presence
As virtual and augmented reality platforms grow, OmniHuman-1 could redefine how people interact in these environments. Instead of relying on generic avatars, users could create highly personalized digital representations of themselves from just a selfie and an audio input. These avatars would mirror real-life gestures and expressions, making virtual meetings, conferences, or social events feel more natural.
This enhanced realism could also improve telepresence applications for businesses. Executives, for example, could deliver presentations or host meetings with fully animated avatars that capture both their likeness and body language, strengthening communication and audience engagement.
Ethical & Legal Questions
The rise of OmniHuman-1 brings both opportunities and challenges. As deepfake technology becomes more sophisticated, concerns about privacy, misinformation, and regulation grow. Here, we examine the ethical and legal implications surrounding AI-generated media.
Deepfake Concerns
The rise of OmniHuman-1 brings both opportunities and challenges. As deepfake technology becomes more sophisticated, concerns about privacy, misinformation, and regulation are becoming more pressing. OmniHuman-1’s ability to generate highly realistic human videos raises the stakes for how AI-generated media is created, distributed, and monitored in both digital and physical spaces.
While the technology promises innovation in entertainment and communication, it also risks being weaponized for deceptive purposes. Addressing these issues requires both legal intervention and stronger societal awareness.
Deepfake Concerns
OmniHuman-1’s advanced capabilities could exacerbate existing concerns around deepfakes, particularly in politics, finance, and online harassment. Politically motivated fake videos, such as those depicting public figures making false statements, have already proven to be powerful tools for disinformation. Similarly, fake endorsement videos have been used to scam investors by impersonating celebrities promoting fraudulent schemes.
Another major concern is the nonconsensual use of someone’s likeness, particularly in explicit or defamatory content. The emotional and psychological toll on victims of such deepfakes can be devastating. Critics emphasize the need for both cultural shifts and legal protections to combat these harms effectively.
Regulatory Environment
Several countries have begun regulating deepfake technology to address its misuse. South Korea has passed laws criminalizing the creation and distribution of harmful deepfakes, with a focus on explicit content. Meanwhile, the European Union has implemented the Artificial Intelligence Act, which includes provisions to limit the spread of deceptive AI-generated content.
However, enforcement remains difficult due to the volume of media produced daily and the rapid evolution of AI capabilities. Detecting deepfakes in real time requires robust technological infrastructure, something many regulatory bodies are still developing. Experts argue that these efforts must be global in scope to be effective, as AI-generated content often transcends borders.
Personal and Social Impacts
Beyond legal concerns, the societal impact of advanced deepfake technology is significant. Public trust in video and audio evidence is already being eroded, with many fearing that it will become increasingly difficult to verify the authenticity of media. This “authenticity crisis” could lead to widespread skepticism of both real and fake content.
For private citizens, the stakes are even higher. Individuals whose likenesses are used in deepfake videos—whether for harassment or fraud—may struggle to clear their name, especially if viral content spreads before corrective actions can be taken. Advocates are calling for clearer consent laws and faster takedown mechanisms to protect victims from such exploitation.
At the same time, public education about the risks and signs of deepfakes is becoming essential. As detection tools improve, fostering digital literacy could help audiences critically evaluate online media, reducing the potential harm of misleading content.
Conclusion
OmniHuman-1 signals a leap forward in AI-driven human animation. By combining a Diffusion Transformer with broad “omni-conditions” training and a massive video dataset, ByteDance has created a tool that can transform a single photograph into a full-motion, realistic video. Its ability to adapt to various body types, aspect ratios, and animation styles makes it an attractive option for filmmakers, marketers, game developers, and many others.
Yet, in step with its promise, OmniHuman-1 also underscores the risks of advanced deepfake technology. As these models become more accessible, questions about authenticity, security, and legal liability multiply. In the coming years, the challenge will be balancing innovative breakthroughs like OmniHuman-1 with the ethical frameworks and detection methods necessary to prevent abuse. For now, it stands as one of the most compelling demonstrations of how far AI-generated video has progressed—and where it may go next.