Artificial intelligence is revolutionizing our world, powering everything from chatbots like ChatGPT to complex scientific research. But behind the convenience and innovation lies a hidden cost—massive data centers that consume huge amounts of energy and water. As more people use AI every day, it becomes critical to understand exactly what these systems require and the environmental consequences of keeping them running.
At the heart of these AI systems are powerful supercomputers that train models like GPT-4. These systems require not only high-performance hardware but also enormous quantities of water to cool the equipment and manage the heat generated during both training and everyday operation. In this article, we’ll break down the ins and outs of AI’s resource consumption, compare it to everyday activities, and look at what companies are doing to balance innovation with sustainability.
Understanding how much water and energy AI consumes isn’t just about numbers—it’s about knowing where we can improve, how we can make technology greener, and how to ensure that the digital future is sustainable for everyone.
From Data Centers to Cooling Systems
AI systems like ChatGPT don’t just “exist” in the cloud—they rely on massive physical infrastructure housed in data centers. These facilities contain thousands of high-performance GPUs (graphics processing units) and specialized AI accelerators designed to handle complex machine-learning tasks. AI models go through two major stages: training and inference.
Training a model like GPT-4 involves analyzing vast amounts of text, requiring weeks of nonstop computation. Inference—the process of generating responses to user queries—is far less demanding but still requires significant computing power at scale.
All of this processing generates an enormous amount of heat. Unlike personal computers that rely on small fans, data centers need industrial-scale cooling to prevent overheating, which can cause performance degradation or hardware failure.
The two primary cooling approaches are air cooling, which uses massive fans and heat exchangers, and liquid cooling, which is far more efficient but also consumes large amounts of water. Microsoft’s AI supercomputer in Iowa, which helped train GPT-4, relies heavily on water cooling, drawing from local rivers to keep servers running at optimal temperatures.
Cooling Methods Explained
- Evaporative Cooling: The most common method, where hot air passes over water, causing evaporation that removes heat. This is highly effective but results in significant water loss.
- Air Cooling: Uses fans and air circulation to dissipate heat. It requires less water but is less efficient for high-density workloads.
- Immersion Cooling: A newer approach where servers are submerged in a special, non-conductive liquid that absorbs heat. This method drastically reduces water use and improves efficiency but is expensive and less widely adopted.
Cooling AI infrastructure isn’t just about efficiency—it’s a major environmental concern. In hot climates, water-cooled data centers can withdraw millions of gallons per day.
On peak summer days in 2022, Microsoft’s Iowa data centers used over 11 million gallons of water, accounting for about 6% of the district’s total water consumption. As AI usage skyrockets, so does the demand for better, more sustainable cooling solutions.
AI’s Environmental Impact
AI’s growing resource consumption has raised concerns, but how does it actually compare to everyday activities? While early estimates suggested that 5–50 ChatGPT queries might use 500 milliliters of water, further research refined this estimate. If we consider only the water directly used for cooling within the data center (excluding water used for electricity generation), the number is closer to 500 milliliters per 300 queries. In practical terms, this means ChatGPT’s direct water consumption is relatively small but can add up as usage increases.
Data from Li, Ren et al. (2023) and the U.S. Census Bureau provide useful comparisons:
- One hamburger requires about 660 gallons of water, equivalent to nearly 200,000 ChatGPT queries.
- One hour of TV in a U.S. household uses about 4 gallons of water, the same as 1,200 ChatGPT queries.
- Leaking pipes in the U.S. waste over 10,500 million gallons of water per day, while ChatGPT’s estimated global daily water consumption is about 3,500 million gallons.
Energy consumption follows a similar pattern. A Google search uses about 0.3 watt-hours, while a ChatGPT query requires around 3 watt-hours—a tenfold increase. However, streaming video for an hour consumes significantly more energy than ChatGPT. Training large AI models like GPT-4 is energy-intensive, sometimes compared to 200 transcontinental flights, but this is a one-time cost spread over billions of queries, reducing the per-query impact.
Research from Shaolei Ren at the University of California, Riverside, alongside disclosures from Microsoft and Google, highlights that while AI has a measurable water and energy footprint, it must be viewed in context. Compared to meat production, inefficient infrastructure, and digital entertainment, AI’s environmental impact is relatively small. The bigger challenge lies in global resource management across industries, not just AI itself.
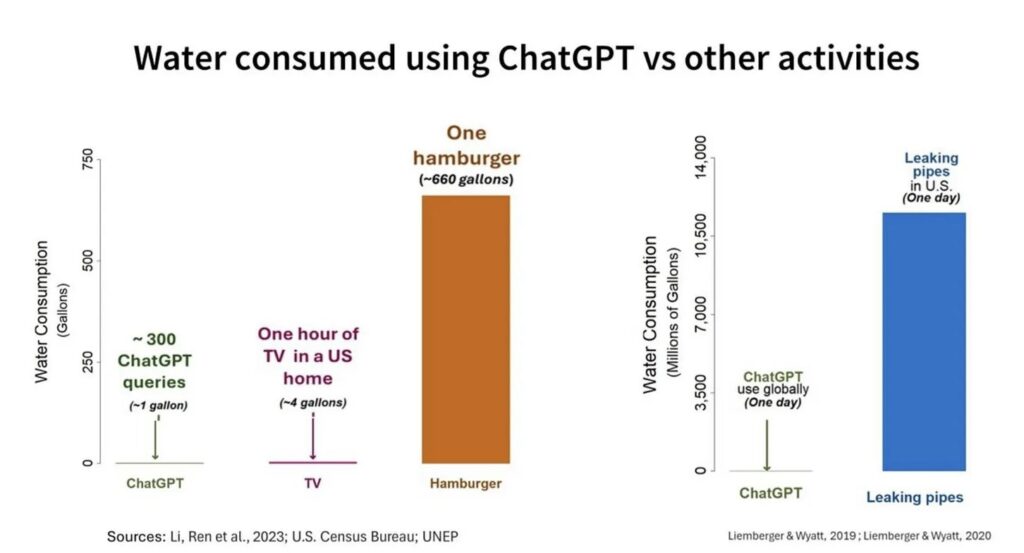
Innovation vs. Sustainability
With the growing demand for AI, tech companies are under increasing pressure to manage their environmental impact. Microsoft, Google, and others are actively researching how to make data centers more efficient, from investing in renewable energy to adopting alternative cooling technologies.
One promising avenue is the development of new cooling solutions that reduce water usage. For example, some companies are exploring immersion cooling—a method that can capture almost 100% of the heat generated by servers without relying on large quantities of water. Other firms are looking at air-based cooling or even using recycled water, particularly in areas where water is a scarce resource.
It’s also important to put AI’s consumption in context. When compared to everyday activities, the additional energy and water used by AI are relatively small. For instance, while ChatGPT might use more energy per search than a Google query, streaming video, which is an integral part of modern life, consumes far more energy overall. By comparing these numbers, we can see that the environmental cost of AI, though significant, is part of a much larger picture of global energy and water use.
Looking ahead, the challenge for tech companies and policymakers is to continue advancing AI technology while adopting practices that are sustainable in the long run. This means investing in green energy, improving data center efficiency, and ensuring that new technologies are developed with the planet in mind.
Future Predictions and Sustainability Goals
As AI adoption grows, so does the urgency for tech companies to mitigate its environmental footprint. Major players like Microsoft, Google, and OpenAI have publicly committed to sustainability goals, including becoming “water positive” by 2030—meaning they will replenish more water than they consume. But how realistic are these targets, and are there already working examples of AI companies successfully reducing water usage?
Can AI Become More Water-Efficient?
AI’s resource consumption has grown exponentially, but so have advancements in cooling technologies and renewable energy integration. Some promising trends include:
- Immersion Cooling: By submerging AI servers in non-conductive liquid, companies can reduce water usage significantly while improving heat dissipation.
- Advanced Heat Recycling: Data centers are beginning to reuse waste heat to provide heating for nearby buildings, reducing overall energy and water consumption.
- AI-Optimized Energy Management: Ironically, AI itself is being used to analyze data center cooling patterns and optimize energy and water efficiency.
Microsoft and Google’s Roadmap
- Microsoft has invested in sustainable water management, including the use of recycled water in cooling systems and plans to run its AI infrastructure on 100% renewable energy by 2025.
- Google is developing AI-powered efficiency tools for its data centers, already claiming to have cut cooling-related energy use by 30%.
- OpenAI has yet to disclose detailed sustainability plans but is reportedly working with Microsoft’s Azure AI supercomputing infrastructure, which is shifting towards greener energy.
Will AI’s Water Consumption Keep Rising?
A critical question remains: Will AI’s efficiency gains outpace its growing demand? While newer AI models are becoming more efficient per query, their increasing complexity and usage may still drive up overall water consumption. For example, more frequent model updates (like the transition from GPT-4 to GPT-5) could offset some of the efficiency improvements.
Ultimately, the challenge isn’t just about reducing AI’s footprint—it’s about integrating it into a broader sustainability strategy. The future of AI will likely depend on balancing technological growth with real-world resource constraints.
Frequently Asked Questions
Below are some of the most common questions people have, with clear, fact-based answers:
How much water does one ChatGPT query really use?
While early estimates suggested about 500 milliliters per 5–50 queries, more detailed research shows that only about 15% of that water flows directly through the data center—roughly 500 milliliters for every 300 queries. The rest is tied to water used in generating the necessary electricity.
What is the difference between training and inference energy use?
Training an AI model like GPT-4 is extremely energy-intensive and is a one-time cost that is spread out over billions of queries. Inference (or answering queries) uses much less energy per query, making the ongoing operational cost relatively modest when amortized over time.
How do data centers keep cool and why is water important?
Data centers use water primarily in cooling systems to dissipate the heat generated by high-density computing hardware. Methods include evaporative cooling, where water absorbs heat through evaporation, as well as newer techniques like immersion cooling. Water is vital because it’s an efficient medium for heat transfer compared to air.
How to reduce the environmental footprint of AI?
Companies are exploring several solutions, such as using renewable energy to power data centers, improving cooling efficiency with innovative methods (like immersion or air cooling), and selecting data center locations with cooler climates or ample water supplies. Ongoing research and sustainability goals (e.g., being “carbon negative” or “water positive” by 2030) are key parts of this effort.
How does AI’s environmental impact compare with other everyday technologies?
Although AI systems like ChatGPT consume more energy per query than a standard Google search, activities like streaming video or online gaming use much more energy overall. In context, the per-user impact of AI is relatively small, but it’s important to continuously improve efficiency as usage scales up.
Conclusion
AI has undeniably transformed the way we live, offering tremendous benefits and new capabilities. However, it also comes with hidden environmental costs—particularly in the form of water and energy consumption. By understanding how data centers work, how cooling systems operate, and how energy is used during both training and inference, we can appreciate the complexities behind these digital tools.
The key takeaway is that while AI does consume significant resources, the industry is actively seeking solutions to make these processes more sustainable. From innovative cooling methods to increased use of renewable energy, the future of AI is being shaped with sustainability in mind. As users and citizens, understanding these issues helps us engage in informed discussions and support initiatives that balance technological advancement with environmental responsibility.
For those searching for answers on “ChatGPT water consumption,” “AI energy usage,” or “how to reduce data center cooling water use,” this guide provides a comprehensive, fact-based look at the challenges—and the potential solutions—behind the digital revolution.